At Musiio, our scalable cloud infrastructure allows us to reliably tag up to 5 million tracks a day with around 50 separate descriptive data points per song (in our standard tags, that includes Genre, Mood, BPM, Key, and more).
That is, to say the least, several truckloads of data. But data on its own is just a bunch of rows and columns. As a catalog owner, artist, or song fund looking to determine the most effective strategy for your music moving forward, how might you put that to use?
A "Data Analysis Recipe"
Here's just one example: using comparison datasets to determine the market fit and overall potential of a piece of music, with a combination of objective and contextual data. It's so simple that you don't even need to be a data scientist to do it.
I should know. I'm not a data scientist, and I did it. All I used was Google Sheets and our Tag API output CSVs.
In order for this "Data Recipe" to work - here are the ingredients you'll need.
- Datasets of music that you know to be successful (If you are a record label or streaming service, using labeled sets of your recently top performing songs will allow you to get an up-to-date picture of what works) For this example, we analyzed songs that did well on Spotify in different countries.
- Tag the song that you want to test for 'Market Fit'
- Compare that song's tags with each of the analyzed and labeled datasets to determine which set it matches most closely with.
Let's take a look at an example of how this works!
Individual Analyzed Country Dataset: Mexico
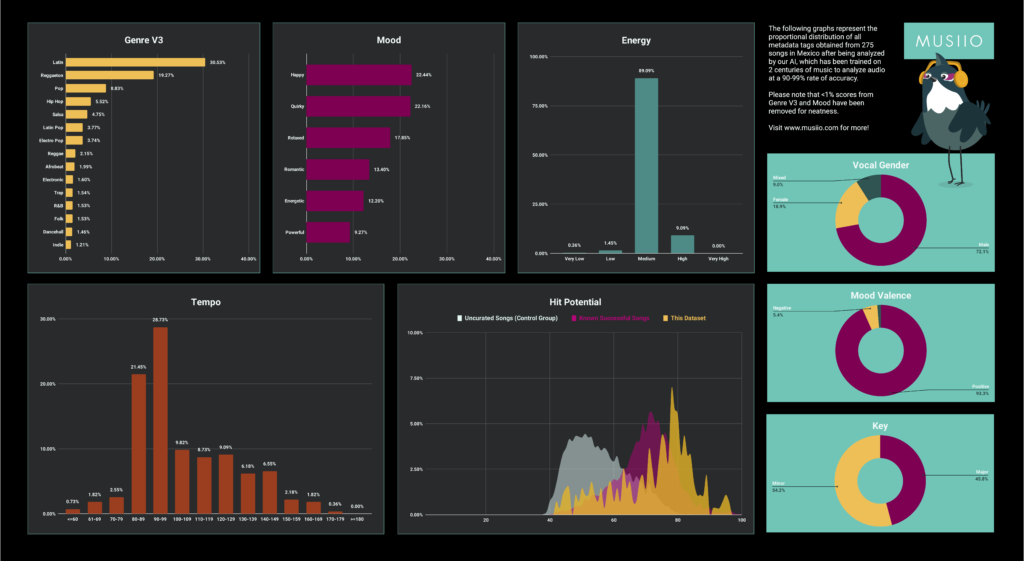
Here is a "Top Line" analysis of Mexico's Spotify Top 200 songs in H2 2020. It has certain very particular characteristics, such as an overwhelming preference for Male Vocals and Positive Mood Valence. Compared to the other 12 sets that were analyzed (including countries from Southeast Asia and Europe), it exhibited a very uniquely overwhelming preference for Latin and Reggaeton music (50% of overall preference), and an unusually small number of dominantly preferred Moods.
In addition, you can also see that its general distribution of Hit Potential scores is even higher than that of generally commercially successful songs, which interestingly correlates with Chartmetric's Trigger Cities concept that successful songs in Trigger Cities (such as those in Mexico) are more likely to go on and do well globally.
Individual Song Matching Example: Cómo Se Siente
https://www.youtube.com/watch?v=ZAW9358S9rY
Here are the tags from our Tag Demo.
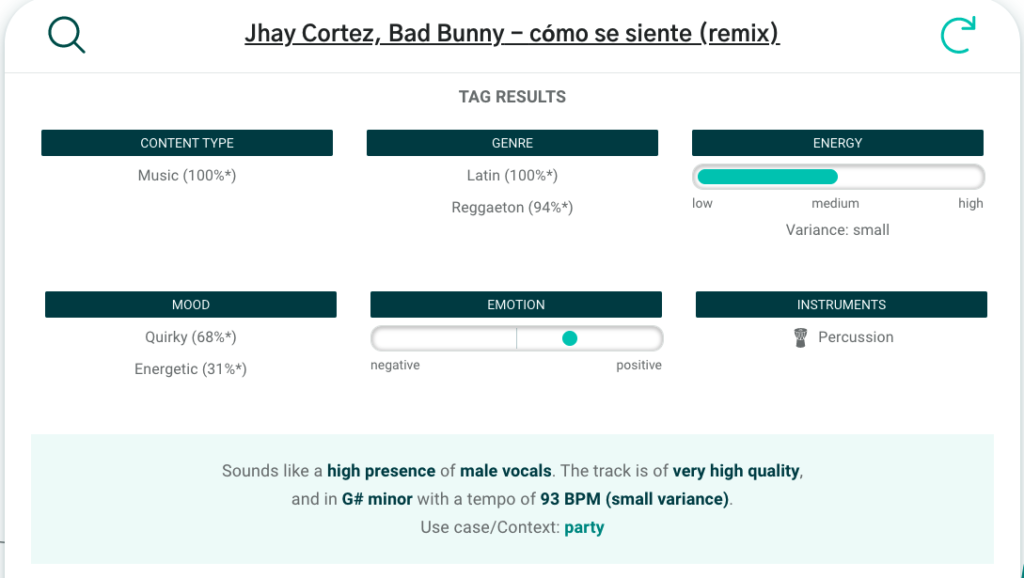
Based on these tags. the following readout shows Mexico is clearly the best match for this track. In the smaller breakdown graphs of individual classifiers, you can see that Mexico matches relatively well across all tags, but emerges an overwhelming winner in Genre, leading to its overall match score of 78.61%.
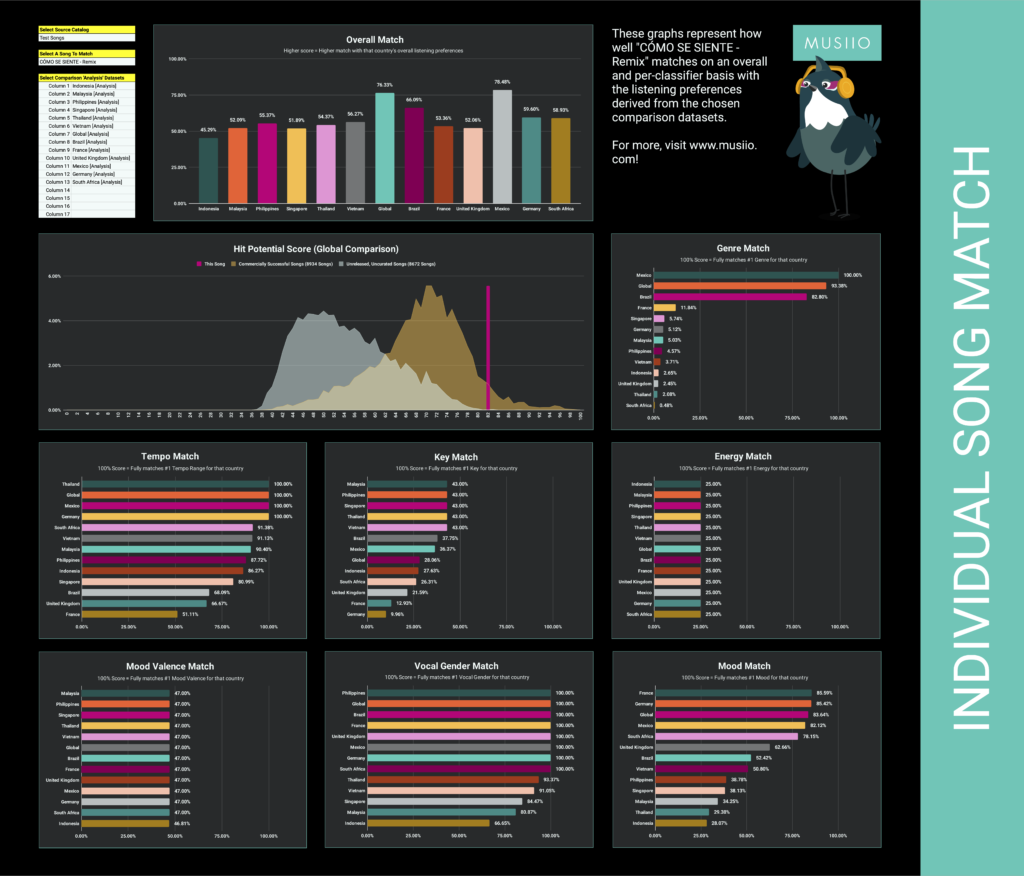
Individual Song Matching Example 2: Be Alright
https://www.youtube.com/watch?v=KRRzgV8CmAQ
Here are the tags from our Tag Demo:
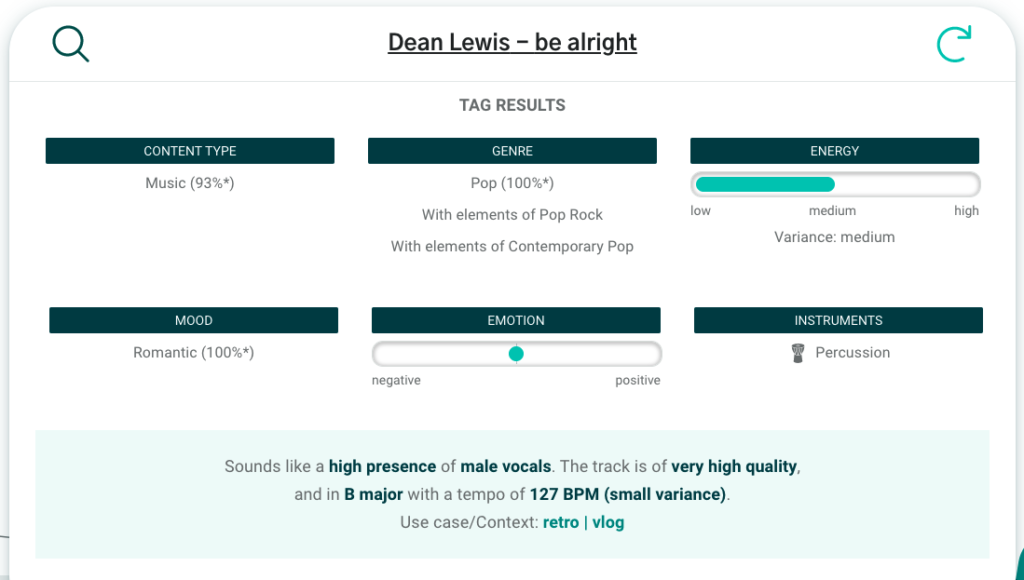
From the following readout it is clear that Mexico emerges as the least compatible country (though the score of 57.3% means it's not a total write off) for this song. The primary mismatched tags are Genre and Tempo, where Mexico comes in dead last.
A note: this song may be interpreted in "half time", in which case the tempo would be 63/64. In this case, it would receive an even worse tempo match score.
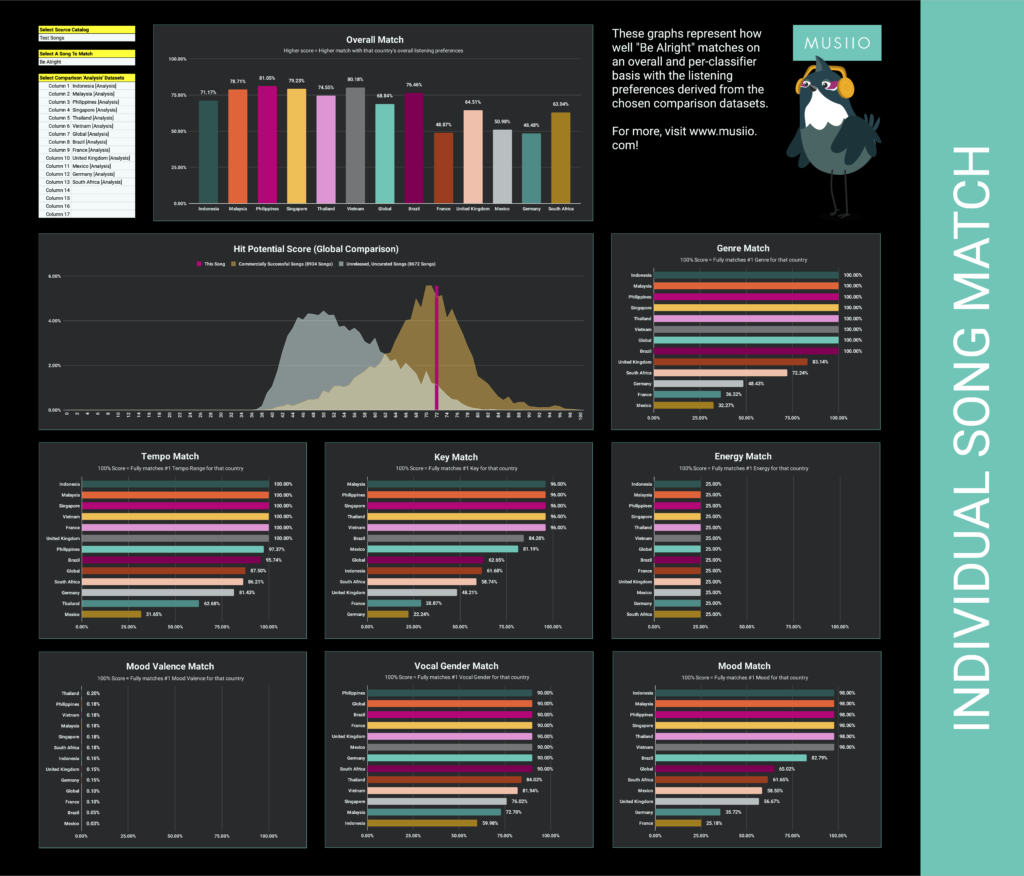
Since we've seen above that Mexico has very particular and pronounced preferences for specific Genres and Tempos, it makes sense then that a "miss is as good as a mile". Some markets are a little more flexible and forgiving, of course.
Let's take a look at the market that had the best match score this time round: the Philippines.
Individual Analyzed Country Dataset: the Philippines
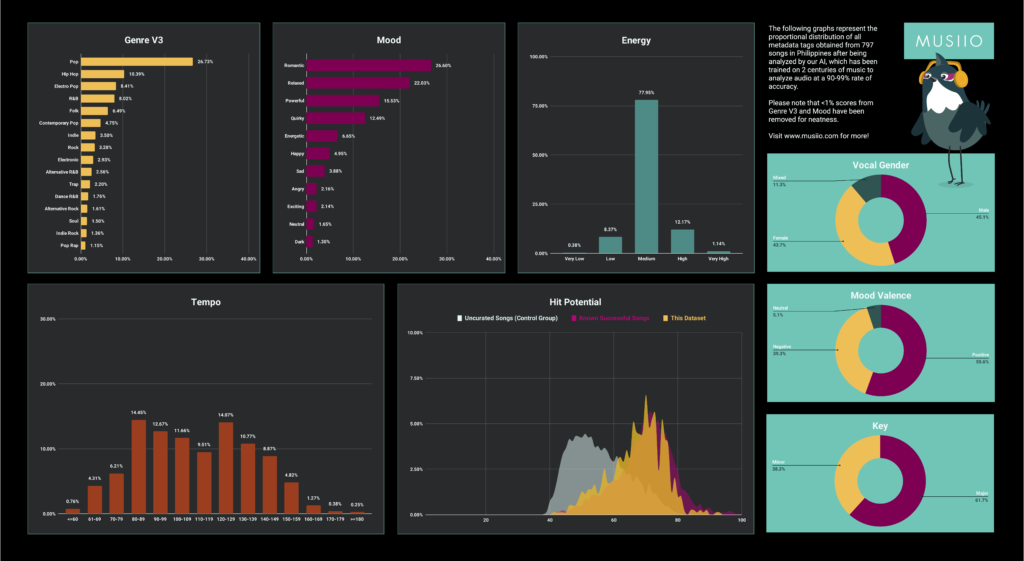
Why exactly did the Philippines perform well here in comparison to Mexico? As you can see in the individual readout above, it matched much more strongly in Genre and Mood, a result shared by all of the Southeast Asian countries. It managed to edge out everyone else in overall match score due to its consistency in matching across all the different tag types.
Conclusion
The technology to enable artists, labels, song funds, and other catalog owners to make data-driven music decisions based on contextual and objective information already exists. Making use of it could help you make a larger volume of accurate and effective decisions more rapidly. What are you waiting for?
If you'd like to work with us to generate similar reports for your use, give us a shout at www.musiio.com!