Creating A Hit
There are many important factors involved in making a song into a hit. Arguably, since we are dealing with music and its listeners, the audio plays a significant part.
So can human beings tell when a song has an “X-Factor”, based purely on the audio?
Many in the A&R business would say yes. Indeed, many commercial music decisions are made based solely on gut feel. But given that approximately 21m+ tracks are released on streaming platforms every year, A&R executives certainly know what it’s like to be inundated in low quality demos.
We’d like to help with that.
So can an AI be trained to help in this process? We’ve trained ours to identify Genre, Mood, and many other aspects of a song with a greater than 90% accuracy. Could we train it to determine, purely by analyzing the audio, whether a song has that “X-Factor?”
Let’s do a little experiment to find out:
The Experiment
Our Hit Potential algorithm listens to tracks and gives them a score between 0-100. The higher the score, the more likely it is to be commercially successful. Or at least, that’s what we claim it does - we’ll let the evidence speak for itself.
We ran 820 recently released songs through the Hit Potential Algorithm, organized in 3 decreasing levels of commercial success.
- Spotify Top 200 (Most Successful/Curated by Global Preferences)
- Spotify New Releases
- User Generated Content (Least Successful/Completely Uncurated)
Results
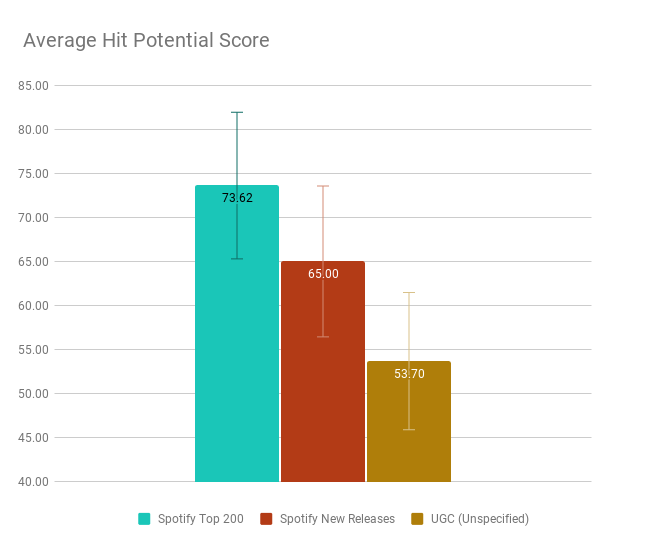
Skip this Paragraph if You're Not a Data Scientist
An analysis of variance (ANOVA) on these scores yielded significant variation among the sets, F(2, 818) = 409, p < .001, where p < 0.05 denotes significance. A post hoc Tukey test showed that all 3 sets differed from each other significantly at p < .001.
These results show a positive correlation between high Hit Potential scores and Commercial Success.
For the Non-Data Scientists
Simply put, the more commercially successful sets of music received higher Hit Potential scores on average, and the less commercially successful sets of music received lower Hit Potential scores on average.
Because our algorithm only listens to the audio, and is unaware of any marketing or branding factors that may lead to commercial success, all of its insights are derived from how a track sounds.
This shows that it can “hear” if a track is more likely to be in a commercially successful set (or if an artist/label needs to work on it a little more before releasing it).
“Minimum" Score For Success?
The following figure shows the percentage of each set that scored >67. Note that with the exception of Spotify Top 200 (which reflects global listening preferences), the other sets are relatively uncurated.

Notably, 85.67% of Spotify Top 200 had >67 Hit Potential score, suggesting that songs with a score of >67 may be more likely to have the potential to eventually make it to this chart.
Conversely, it shows that songs with <67 Hit Potential score are highly unlikely to ever make it to this list.This may be a useful benchmark for artists and labels to use in pre-production, to improve songs before release.
Consider the implications of using this during a songwriting or production session to determine if something requires more work or if changing it further might actually be detrimental.
Conclusion
In conclusion, there is a clear, analyzable difference between commercially successful and less successful tracks based on audio alone. The data shows that our Hit Potential Algorithm is able to understand and quantify this difference.
There are things machines are good at, and there are things humans are good at.
Going through a million songs in a day? That’s a machine’s job.
Looking at 100 songs and deciding the best one based on instinct and subtle nuances?
Now, that’s the human being’s job. Welcome to the age of AI & R.